When Instagram switched from showing posts in reverse chronological order in 2016, it created a new precedent of social media companies no longer relying on their users to define the content they wished to see. Instead, these organizations implemented complex machine learning algorithms to choose what people would see with the intent of keeping consumers on their respective platforms for the longest amount of time possible. From a solely business perspective, this change in how media companies handle users led to an enormous increase in ad revenue, as the more time someone spends on Instagram, or any other network, results in more ads being displayed and more opportunities for clicks. In the case of Instagram, many consumers were outraged and believed that they could no longer define what posts they were able to see, and the idea of a computer managing that decision seemed unnerving. But these algorithms are incredibly effective, and over time continually improve by learning more about users’ interests and the type of content that would keep their eyes glued to the screen. While the negative feedback directed towards Instagram’s change has died down, many negative consequences have surfaced about how computers can alter people’s opinions and cause their ideologies to move farther towards extreme beliefs.
Even without the biases of programmers, these algorithms have a strong potential to be dangerous. After conducting an analysis of social media behavior, researchers at the University College London found that social networks “enable confirmation bias on large scales by empowering individuals to self-select narratives they want to be exposed to” (Livan, Cornell University, https://arxiv.org/abs/1808.08524). Although critics may argue that before these algorithms were employed users still had the ability to choose what kinds of content they viewed, there is still a convincing case to be made that computers curating content removes the control a person has over determining what sources of information they find trustworthy or valuable. Since these algorithms are programmed with one goal in mind, the accuracy of information plays a small role in whether or not an article or post will be recommended. For example, if a news article is considered to be more likely to retain a user, it will likely be recommended regardless of its accuracy.
YouTube is a great platform to showcase how information presented to individuals can easily be biased and misleading. The company uses a “Deep Neural Network” to recommend videos, which trains itself by taking in hundreds of metrics on user behavior and testing to see what causes each viewer to continue watching. The figure below is a simplified explanation of how videos are selected for each person.
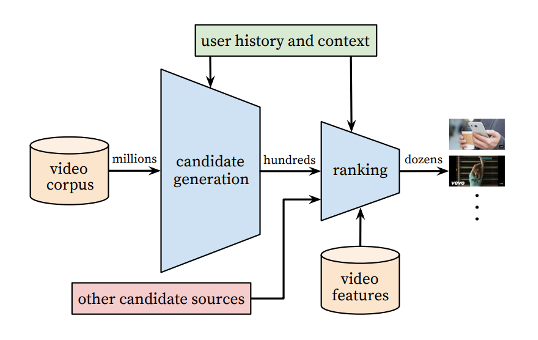
(“Deep Neural Networks for YouTube Recommendations,” Google, static.googleusercontent.com/media/research.google.com/en//pubs/archive/45530.pdf)
Given that millions of people look to YouTube as their primary source of political information, this graph is chilling. By merely analyzing “user history and context,” the platform recommends videos exclusively on someone’s’ preexisting views and current ideology. This blatant disregard for factual and diverse sources of information will clearly lead to furthering a person’s viewpoints, regardless of whether or not their views are harmful to others. To fully understand how this system works in practice, I conducted an experiment. By searching the phrase “crazy liberals” on YouTube and clicking on five videos from conservative channels, I checked to see what recommendations showed up at the end of the last video, which is pictured below.
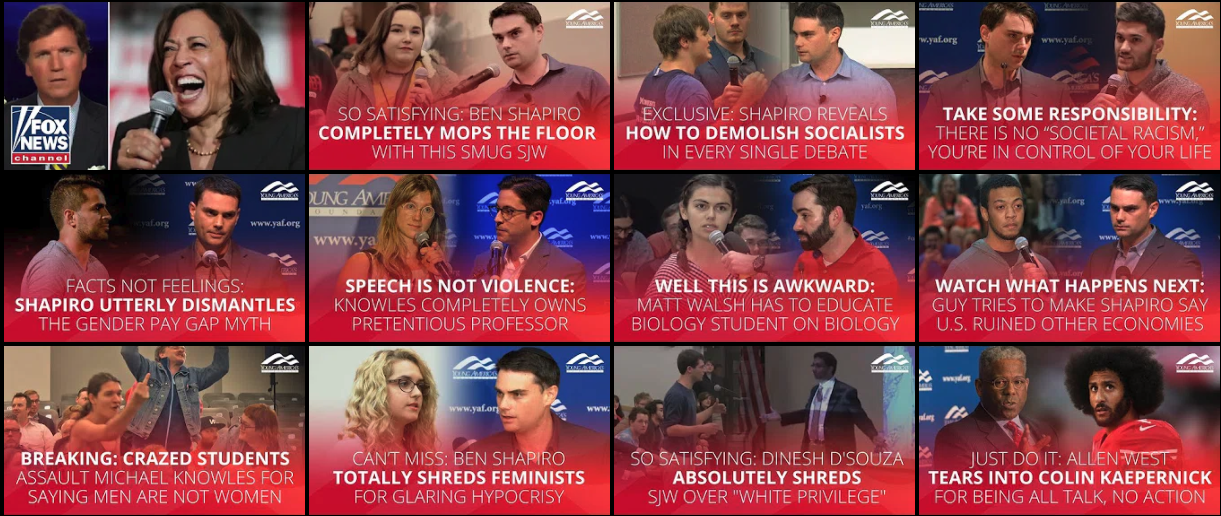
(YouTube.com recommendations after searching for conservative videos)
Every single recommendation, based on my short watch history, is sensationalist conservative media. This is not an attempt to argue that this only happens for right-wing videos; it certainly happens on the other side of the aisle too. It is painfully easy for YouTube’s algorithm to identify a persons’ beliefs and recommend videos that match their viewpoints while disregarding anything that may provide counter-arguments or different perspectives.
YouTube is simply one example of how almost all social networks organize and display content to its users, and how there is a complete disregard for diversity of ideologies. Instagram, Twitter, Facebook, and TikTok all use similar algorithms to recommend users and posts, all for one simple reason. The more time a person spends on a social media platform, the more revenue a company makes. These companies were never intended to be places where users could learn about diverse viewpoints. The users are the people who keep the lights on at all social networks; the information is not the product, the users are the product to advertisers. As long as the only incentive is to keep people’s eyes, this is never going to change.
-Andre Hebra